Agentic AI vs. Generative AI: What You Need to Know
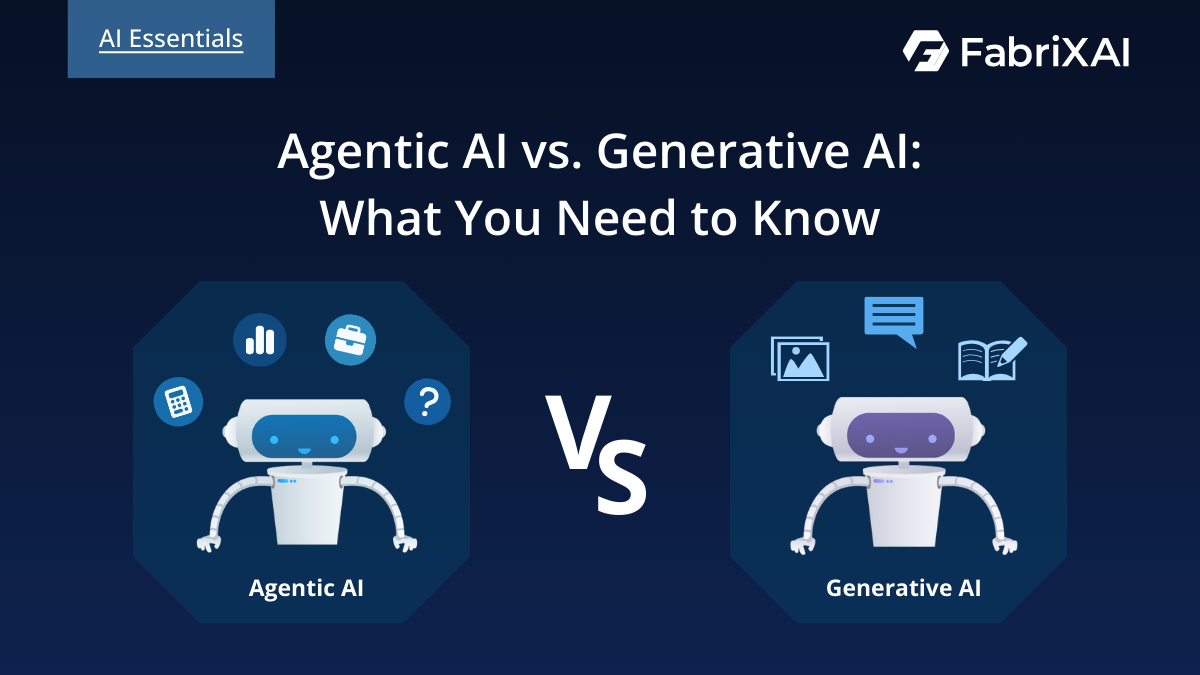
Understanding Agentic AI and Generative AI
Imagine you’re launching a new marketing campaign for a tech startup. You need to create engaging content that resonates with your audience, but you also want to optimize your advertisement placements and target the right demographics. Should you turn to Generative AI (Gen AI) to craft compelling copy and visuals, or rely on Agentic AI to analyze data and make strategic decisions on ad placements?
In today’s rapidly evolving technology landscape, both agentic AI and Gen AI are making significant impacts on enterprises. Understanding their differences can help you choose the right tool for your campaign. This blog post will explore what sets them apart, their applications, and what the future holds for each.
Discovering Agentic AI
Agentic AI refers to autonomous systems designed to perform tasks independently. These intelligent agents can make decisions, plan, and act without human intervention, adapting to new situations effectively. Check out on Introduction to Agentic AI and Agentic Workflow for more information.
Exploring Generative AI
Generative AI (Gen AI) is a groundbreaking technology that creates new content by learning from existing data patterns. It excels in producing human-like outputs, making it invaluable in creative industries.
1. Key Capabilities of Generative AI
Gen AI models can create diverse content, including text, images, music, and videos. By analyzing large datasets, they learn to mimic various styles, producing original work that often rivals human creativity. This capability is vital in industries like marketing, entertainment, and design, where unique content is increasingly in demand.
Additionally, Gen AI personalizes content to match user preferences, enhancing engagement and satisfaction. This makes it a valuable tool for businesses looking to connect with their audiences. As technology advances, the potential applications of Gen AI will likely grow, paving the way for new innovations and creative opportunities.
2. How Generative AI Works
These models, such as GPT-4 and DALL-E, use neural networks to analyze input data, identify patterns, and generate coherent outputs. They leverage deep learning techniques to refine their creativity and produce high-quality results.
Gen AI is transforming creativity by providing innovative tools that enhance productivity and open new possibilities across various fields.
Agentic AI vs Generative AI: A Comparative Analysis

1. Autonomy vs. Creativity
Agentic AI emphasizes autonomy and decision-making. It functions independently, making real-time decisions and interacting with its environment to achieve specific goals. Examples include autonomous vehicles and intelligent personal assistants.
On the other hand, Generative AI excels in creativity. It generates new content like text, images, or music by learning patterns from existing data. Tools like GPT-4o and DALL-E are prime examples, producing human-like text and art.
2. Goal-Oriented vs. Output-Oriented
Agentic AI is goal-oriented, designed to achieve specific outcomes. It navigates complex systems and makes decisions to reach defined objectives, such as optimizing a smart home or managing a robotic process.
Generative AI, however, is output-oriented. It focuses on producing creative outputs based on given inputs, without making independent decisions. Its strength lies in content creation, transforming industries like writing, design, and entertainment.
3. Interaction with Environment
Agentic AI engages actively with its environment, allowing real-time adaptation and decision-making to achieve specific goals. For example, in smart homes, it optimizes energy use by adjusting settings based on occupancy patterns.
Generative AI primarily generates content from existing data and lacks dynamic interaction with its surroundings. While it creates impressive outputs like articles and images, it does not adapt to new information or user feedback.
4. Use of Tools and Resources
Agentic AI uses various tools and APIs to autonomously plan and execute tasks, enhancing efficiency through real-time responses. For instance, in supply chain management, it analyzes inventory and shipping schedules to optimize resource allocation.
In contrast, Generative AI relies on learned data to produce creative content without the use of external tools. While this approach fosters creativity, it limits application scope, as it cannot refine outputs through real-world interaction.
5. Learning and Adaptation
Agentic AI continuously learns from feedback, enhancing its decision-making and performance in dynamic environments. For instance, in customer service, it adjusts responses based on user interactions, boosting satisfaction and personalization.
Conversely, Generative AI learns from datasets but does not adapt based on real-world feedback. This results in static outputs that may become less relevant over time, lacking the flexibility of continuous learning.
6. Application Spectrum
Agentic AI finds applications in fields requiring autonomy and decision-making, such as:
- Robotics: For autonomous operations and decision-making.
- Autonomous Vehicles: Navigating and making real-time driving decisions.
- Smart Assistants: Managing tasks and interactions independently.
Generative AI is prevalent in creative industries, utilized for:
- Content Creation: Writing, art, and music production.
- Design: Generating realistic images and videos.
- Virtual Worlds: Creating immersive environments in gaming and simulations.
Best Practices for Implementing Agentic AI
Implementing Agentic AI effectively requires careful consideration and adherence to best practices to maximize its benefits. Here are some key strategies:
- Begin with specific, focused use cases: Target clear applications to streamline development and measure success.
- Ensure strong data governance and security: Implement robust data management and security measures to protect sensitive information.
- Maintain human oversight in critical decision processes: Keep humans involved in significant decisions to ensure balanced and ethical outcomes.
- Invest in continuous training and development: Regularly update the AI model with new data to enhance its learning and effectiveness.
- Foster cross-functional collaboration: Engage diverse teams to ensure comprehensive understanding of organizational needs.
- Monitor and evaluate performance regularly: Use key performance indicators (KPIs) to track effectiveness and guide improvements.
- Develop a clear ethical framework: Establish guidelines to address biases and ensure fairness in decision-making.
The Future of Agentic AI and Generative AI
1. Agentic AI
As technology continues to advance, Agentic AI is poised to transform various sectors and workflows significantly.
- Enhanced Decision-Making:
- Agentic AI will increasingly autonomously handle complex decisions, improving efficiency and effectiveness across various domains.
- Integration with IoT:
- Seamless integration with Internet of Things (IoT) devices will create smarter, interconnected environments that optimize resource use and enhance user experiences.
- Advanced Robotics:
- The development of more sophisticated robots will expand capabilities in sectors like manufacturing, healthcare, and logistics, enabling greater automation and precision.
- Personalized User Interactions:
- Agentic AI will leverage data to offer highly personalized experiences, adapting to individual preferences and needs in real-time.
2. Generative AI
In parallel, Generative AI is set to redefine creative processes and content generation in innovative ways.
- Contextual Creativity:
- Generative AI will evolve to create content that adapts to real-time contexts, enhancing relevance and engagement.
- Improved Personalization:
- Advanced algorithms will enable Generative AI to produce highly personalized outputs, catering to individual preferences and needs.
- Ethical Frameworks:
- The development of robust ethical guidelines will ensure responsible use, minimizing biases and promoting fairness in content generation.
- Collaboration with Humans:
- Generative AI will increasingly serve as a collaborative partner, enhancing creative processes and allowing for more innovative outcomes through human-AI synergy.
Conclusion
Agentic AI and Generative AI are set to revolutionize industries with their unique capabilities. Understanding their differences and potential helps us harness their power to drive innovation forward. As technology continues to advance, these AI systems will play an increasingly crucial role in shaping our world.
By exploring these technologies, businesses can better position themselves for a future where AI is integral to success.